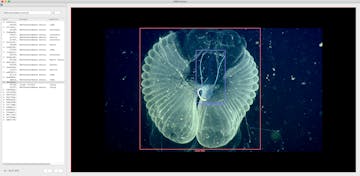
Annotation and localization of a giant larvacean, Bathochordaeus mcnutti, as it leaves its mucus house. These data are then added to the FathomNet database, which will be used to train machine learning models to automate the detection and classification of objects in underwater imagery and video. These augmented data are generated using MBARI’s VARS Localizer software tool.
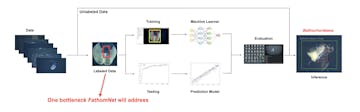
Diagram illustrating the bottleneck that FathomNet aims to address, which is a lack of labeled (or annotated and localized) image data. Labeled data are required to train and test machine learning models that can then be subsequently used on unlabeled data.
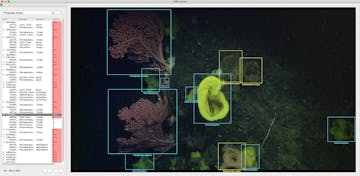
Existing VARS annotations contain information about what objects are in the frame, but excludes information about where it is in the frame. Localization, or the process of associating position data by generating bounding boxes, in addition to object annotations, are required to train machine learning models. Tools to localize objects within VARS image and video data, like VARS Localizer, have been developed to address this need.
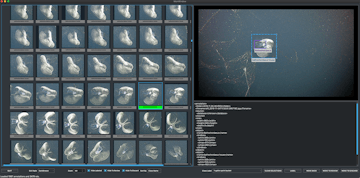
MBARI’s software tool, the ML-tracking GUI, is used to quickly validate machine-generated annotation and localization proposals from analyzed ROV dive footage. Tools like these have been developed by MBARI engineers to speed up the data curation process to generate machine learning training data and validate machine learning models.
This video shows machine learning proposals of the giant larvacean species, Bathochordaeus mcnutti, and its mucous inner filter. To perform this automated tracking and classification, a machine learning model was first trained using localized images of this species from other observations contained within MBARI’s VARS database and aggregated into FathomNet. Models like these are being used as part of the ML-Tracking project, which aims to integrate machine learning with underwater vehicle control algorithms to automate the acquisition and long-duration tracking of animals in situ.