In order to manage impacts from climate change and other threats, researchers urgently need to learn more about the ocean’s inhabitants, ecosystems, and processes. As scientists and engineers develop advanced robotics that can visualize marine life and environments to monitor changes in the ocean’s health, they face a fundamental problem: The collection of images and video, or visual data, vastly exceeds researchers’ capacity for analysis.
A collaborative effort between MBARI and other research institutions is leveraging the power of artificial intelligence and machine learning to accelerate efforts to study the ocean.
FathomNet—co-founded by MBARI, Ocean Discovery League, and CVision AI—is an open-source image database that uses state-of-the-art data processing algorithms to help process the backlog of visual data. Using artificial intelligence and machine learning will alleviate the bottleneck for analyzing underwater imagery and accelerate important research around ocean health.
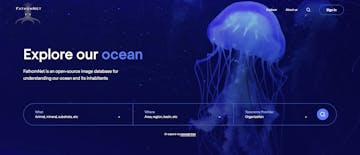
FathomNet is an open-source image database that uses state-of-the-art data processing algorithms to help process the backlog of visual data. Image courtesy of FathomNet
Recent advances in machine learning enable fast, sophisticated analysis of visual data, but the use of artificial intelligence in ocean research has been limited by the lack of a standard set of existing images that could be used to train algorithms to recognize and catalog underwater objects and life. FathomNet addresses this need by aggregating images from multiple sources to create a publicly available, expertly curated underwater image database.
To jumpstart the collection, FathomNet is seeded with data that represents a subset of MBARI’s data repository, as well as assets from National Geographic and NOAA.
Over the past 35 years, MBARI has recorded nearly 28,000 hours of deep-sea video and collected more than 1 million deep-sea images. This trove of visual data has been annotated in detail by research technicians in MBARI’s Video Lab, who are experts in the field of visual taxonomy. MBARI’s video archive includes approximately 8.2 million annotations that record observations of animals, habitats, and objects. This rich dataset is an invaluable resource for researchers at the institute and collaborators around the world.
“A big ocean needs big data. Researchers are collecting large quantities of visual data to observe life in the ocean. How can we possibly process all this information without automation? Machine learning provides an exciting pathway forward.” —MBARI Principal Engineer Kakani Katija
The National Geographic Society’s Exploration Technology Lab has been deploying versions of its autonomous benthic lander platform, the Deep Sea Camera System, since 2010, collecting more than 1,000 hours of video data from locations in all ocean basins and in a variety of marine habitats. These videos have subsequently been ingested into CVision AI’s cloud-based collaborative analysis platform and annotated by subject-matter specialists at the University of Hawaii’s Deep-Sea Fish Ecology Lab and OceansTurn.
National Oceanic and Atmospheric Administration (NOAA) Ocean Exploration began collecting video data with a dual remotely operated vehicle system aboard NOAA Ship Okeanos Explorer in 2010. More than 271 terabytes are archived and publicly accessible from the NOAA National Centers for Environmental Information (NCEI). NOAA Ocean Exploration originally crowd-sourced annotations through volunteer participating scientists, and began supporting expert taxonomists in 2015 to more thoroughly annotate collected video.
With data from MBARI and the other collaborators as the backbone, the team hopes FathomNet can help accelerate ocean research at a time when understanding the ocean is more important than ever. MBARI launched a pilot program to use FathomNet-trained machine-learning models to annotate video captured by remotely operated underwater vehicles (ROVs). Using AI algorithms reduced human effort by 81 percent and increased the labeling rate tenfold. As an open-source web-based resource, other institutions can contribute to and use FathomNet instead of employing traditional, resource-intensive efforts to process and analyze visual data.
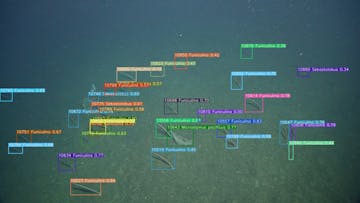
FathomNet aggregates images from MBARI and other institutions to create a publicly available, expertly curated underwater image training database. FathomNet will help unlock the power of artificial intelligence to accelerate the processing of ocean imagery. Image: © 2020 MBARI
Machine-learning models trained with FathomNet data also have the potential to revolutionize ocean exploration and monitoring. For example, outfitting robotic vehicles with cameras and improved machine-learning algorithms is already enabling automated search and tracking of marine animals and other underwater objects.
As of February 2023, FathomNet contained 90,086 images, representing 181,859 localizations from 81 separate collections for 2,243 concepts, with additional contributions ongoing. FathomNet aims to obtain 1,000 independent observations for more than 200,000 animal species in diverse poses and imaging conditions—eventually more than 200 million total observations. For FathomNet to reach its intended goals, significant community engagement—including high-quality contributions across a wide range of groups and individuals—and broad database utilization will be needed.
While FathomNet is a web-based platform built on an API where people can download labeled data to train novel algorithms, it will also serve as a community where ocean explorers and enthusiasts from all backgrounds can contribute their knowledge and expertise and help solve challenges related to ocean visual data that are impossible without widespread engagement.
To join the FathomNet community, visit fathomnet.org and follow @FathomNet on Twitter.
FathomNet forms the foundation for a larger initiative to use AI for ocean exploration.
Last fall, the National Science Foundation (NSF) awarded MBARI $5 million for Ocean Vision AI, a new program that leverages artificial intelligence and machine learning to accelerate processing of—and access to—ocean video and imagery to enable effective marine stewardship.
Ocean Vision AI combines the expertise of MBARI, the Central and Northern California Ocean Observing System (CeNCOOS), Climate Change AI, CVision AI, Ocean Discovery League, and Purdue University, to create a machine-learning platform that will aid the processing of underwater visual data with a globally integrated network of services, tools, and diverse community of users. Multidisciplinary collaborators and supporting team members that include data scientists, oceanographers, game developers, and human-computer interaction experts will streamline access and analysis of ocean visual data to promote effective marine stewardship. Ocean Vision AI’s scope goes beyond data aggregation and processing pipelines. This project will also develop a video game to cultivate a community of ocean enthusiasts that can help improve machine-learning models and contribute directly to ocean exploration and discovery.
Analyzing visual data—particularly data with complex scenes and organisms that require expert classifications—is a resource-intensive process that is not scalable in its current form. As more visual data are collected, the ocean community faces a data analysis backlog that artificial intelligence can help solve.
The new Ocean Vision AI program will:
Provide a central hub for groups conducting research using underwater imaging, artificial intelligence, and open data;
Create data pipelines for image and video data repositories;
Provide project tools for coordination;
Leverage public participation and engagement via game development; and
Generate data products shared with researchers and other open-data repositories.
Ocean Vision AI also seeks to build a global community, from enthusiasts to experts, around underwater visual data akin to an iNaturalist or eBird for the ocean.
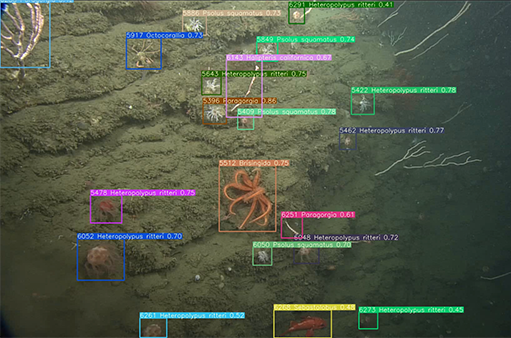
Advancements in machine learning and artificial
intelligence can help process the deluge of data researchers are
collecting about the ocean, but still require human intervention to
train, evaluate, verify, and improve the performance of these
algorithms. Ocean Vision AI will develop game-based human annotation
pipelines to engage a broader audience. A video game will teach casual
gamers about the ocean while improving machine-learning models and
expanding annotated datasets.
A long-term goal of Ocean Vision AI is not only to accelerate ocean discoveries in video and imagery but also to provide these findings and the tools used to support them to a much wider section of the ocean community. Researchers studying ways to manage or conserve ocean resources, and anyone interested in what happens below the ocean surface, will be able to access the resulting verified annotations and trained algorithms via open-source platforms like FathomNet. In the future, this new technology has the potential to usher in real-time processing of ocean imagery, scaling up our observation capabilities using current and future robotic technologies.
Technology innovations like Ocean Vision AI and FathomNet are critical to accelerating the exploration of the ocean and addressing the long-standing challenge of enabling large-scale biological observations in the marine environment so we can sustainably manage and care for this incredible, shared resource.